BigQuery on Center Stage for Google Analytics
For those using Google Analytics, the term BigQuery might sound familiar. That is likely because it is one of the most powerful integrations the paid version of Google Analytics 360 (Universal) has to offer.
Google Analytics 4 (GA4), the newest iteration of Google Analytics, will make this integration available to all. This integration will be something advanced users of Google Analytics will want to understand – for better or for worse.
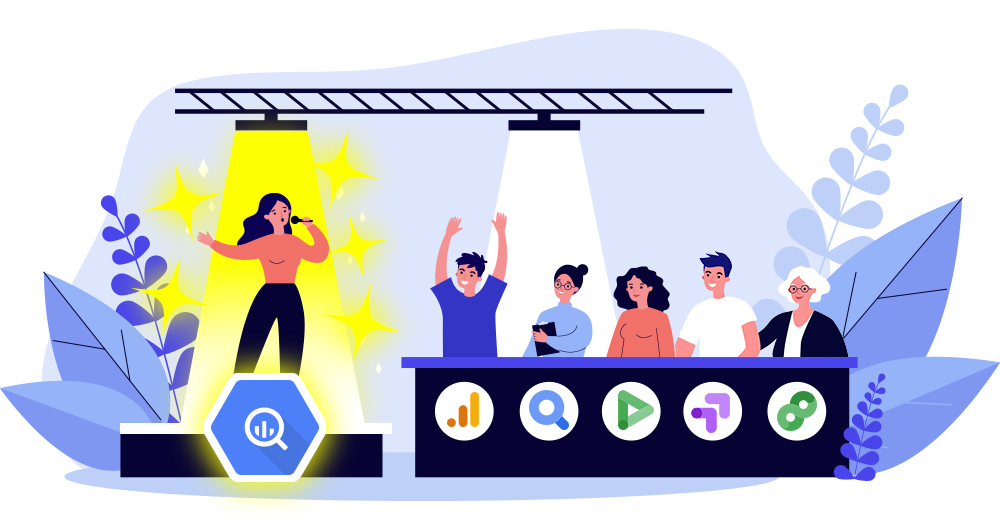
What is BigQuery
BigQuery is one of Google Cloud Platform’s most powerful offerings when it comes to data analytics: a fully managed enterprise data warehouse built-in features like machine learning, geospatial analysis, and business intelligence.
Many people confuse BigQuery as an extension or feature of Google Analytics. It is not. It is technically not even part of the Google Marketing Platform. BigQuery is part of the Google Cloud Platform suite of services, completely independent from Google Analytics.
The reason why BigQuery is so pervasive in most advanced Google Analytics conversations is because GA can easily integrate with BigQuery and export a copy of the raw data automatically. Once this data is in BigQuery it is yours to use as you see fit.
Equally important for analytics purposes, Google Analytics is not the only service that integrates with BigQuery. Take a look at the list below of some of the Google products that have similar exporting features:
- Campaign Manager
- Google Ad Manager
- Google Ads
- Google Merchant Center (beta)
- Google Play
- Search Ads 360
- YouTube – Channel Reports
- YouTube – Content Owner Reports
Why BigQuery Is Now More Important (GA4)
For the better part of the last two years we have heard how Google Analytics 4 is rethinking key components of Google Analytics as we know it – a new data schema, new reports, better machine learning capabilities and, what we are exploring on this post, making various integrations with other Google products free for everyone.
Personally, the ability to export Google Analytics raw data to BigQuery has been one of my favourite features. In the case of Google Analytics Universal, many organizations with resources to afford the paid version of the tool did so with this feature in mind.
With GA Universal, however, the business challenges that required for BigQuery were relatively advanced. In contrast, GA4 brings more basic business challenges where BigQuery becomes close to a requirement.
To be be clear, GA4 enabling BigQuery integration for everyone is, great! However, it is important to acknowledge that extracting and visualizing insights from data in BigQuery is not easy. Handling data from BigQuery requires SQL knowledge, and above average SQL knowledge if we are talking abut Google Analytics data. Then there is creating visualization, a completely different skill.
Many organizations don’t have these skills at hand or the budget to hire them, which may put many organizations at a disadvantage.
New Analytics Business Challenges Requiring BigQuery
Let’s explore three of the most common business challenges in the GA4 transition where BigQuery becomes a blessing, and sometimes also a curse.
Unifying Trends from GA Universal and GA4 for Time Comparisons
As it is constantly advertised, Google Analytics Universal properties will stop processing data July 1, 2023 (October 1, 2023 for Analytics 360 properties). In other words, GA Universal reports will stop collecting data. All trends will stop. Fin. The End.
This poses a challenge for those teams that rely on comparisons across time in the GA user interface to make business decision. With GA Universal properties stopping collection and GA4 property collecting from a relatively recent point, how do you compare across time between the two? You cannot, at least not in Google Analytics.
The solution relies in taking your data out of Google Analytics into somewhere else, and the natural place to first consider is BigQuery.
GA4 will be exporting data to BigQuery automatically, which will allow both sets of data to live in the same data warehouse. The schemas will be different, the analytics engine is a little different, so there won’t be a 1:1 relation between both data sets, but it will be possible to create some semblance of continuity for the KPIs that matter.
GA4 Data Retention is Only 14 Months (for standard properties)
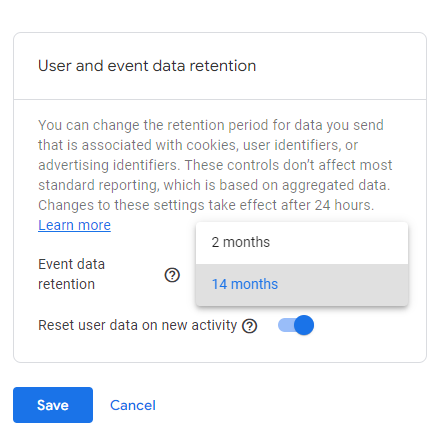
You read that right. Unless you have the paid version of Google Analytics, GA4 will only retain data for 14 months. As you can imagine, many organizations that use the free version of Google Analytics will want time comparisons beyond 14 months. Again, pulling data out of Google Analytics to somewhere that does not expire, like (you guessed it) BigQuery, is a natural consideration.
This is both a blessing and a curse. It’s a blessing because you get a level of detail that is beyond what you can do in the Google Analytics native reports. And it is a curse because this data is no rubbish unless you have the skills to query the right data and visualize it.
Exploration Reports are Often Sampled
GA4 did a great job at reimagining the native reports available to the user. From many reports that were often unused, they went to a few reports that would hopefully be useful enough to address most business insights. This allowed for a simpler and more decluttered navigation menu. On top of that they enabled the “Explore” reports to everyone, which were exclusive to the paid version of Google Analytics Universal.
The “Explore” reports are super flexible and beyond what was possible in GA Universal. In this sense, these reports become a typical go-to solution for many business questions. The problem, however, is that these reports are often sampled.
Many teams rather use raw data in BigQuery to avoid sampling and get the insights with 100% of the data in its calculations.
This, while flexible, creates two source of data for reporting: Google Analytics (anyone can report) and BigQuery (requires expertise to extract and visualize data).
It is worth mentioning that Google Analytics does have features that are difficult to replicate in BigQuery – i.e. predictive metrics generated by machine learning, suggested audiences, etc. In this sense, teams need to consider when to use BigQuery and when to use Google Analytics for reporting and insight exploration.
Hello Other Data and Analysis Beyond Google Analytics
One benefit of this integration that has no drawbacks is the ability to join data with other data to create richer audiences and insights that can be used in an organization marketing strategy beyond Google Analytics.
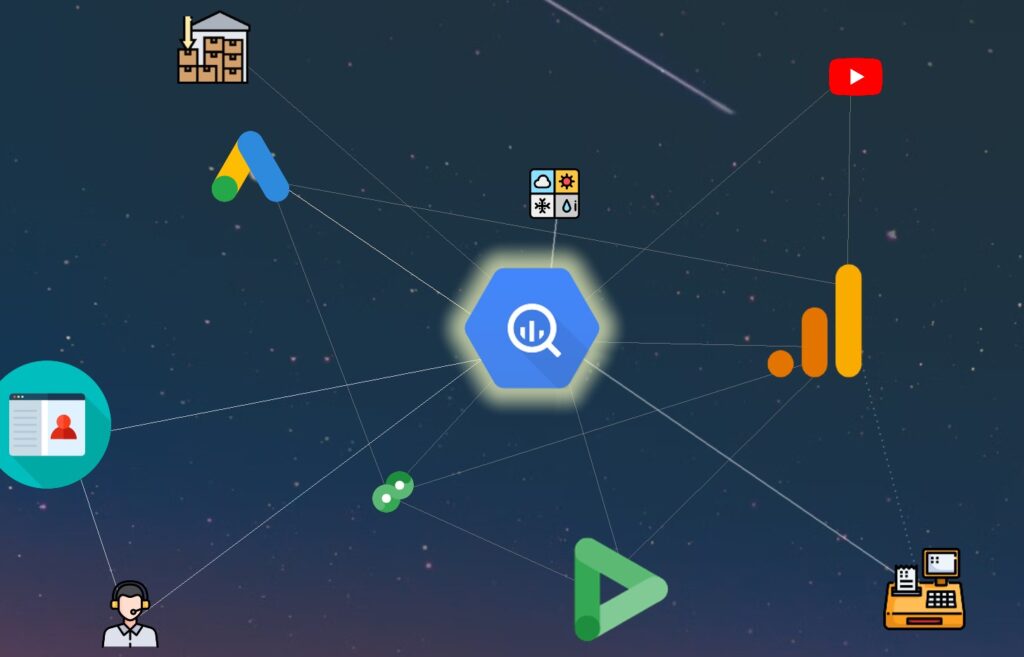
Many organizations with raw data in BigQuery opt to join data from marketing campaigns, loyalty programs, Customer Relationship Management platforms and a variety of other useful data creating a more comprehensive view of customer cohorts.
Even more exciting, BigQuery has built in machine learning models that can tease out insights that are almost impossible to extract by regular analysis. These include supervised and unsupervised learning algorithms that could reveal things like customer lifetime value, relationship between products, and cohorts of valuable customers.
All these benefits take a more human resources and cost, but the tools are there, waiting to provide an advantage to those who can invest on it.
In Conclusion, Learn BigQuery’s Role in Your New Analytics Setup
Unlike GA Universal which has been able to support many type of organizations, big and small, GA4 positions itself as a part of a bigger ecosystem that relies a bit more in other services for any implementation that goes beyond the basics.
It is essential those using Google Analytics moving forward understand the role BigQuery and the other services available as it pertains to their business strategy and objective.
Some organizations may find themselves achieving their business goals solely with Google Analytics; some organizations will encounter some frustration. The key will be in the ability to understand the new landscape along with its advantages and limitations.